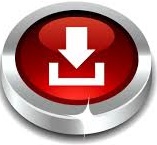
SIFT and SURF are recent key-point or interest point detector algorithms but a point to note is that SURF is patented and its commercial usage restricted. Like Moravec, they needed a method to match corresponding points in consecutive image frames, but were interested in tracking both corners and edges between frames.

They needed it as a processing step to build interpretations of a robot's environment based on image sequences. Harris and Stephens improved upon Moravec's corner detector by considering the differential of the corner score with respect to direction directly. However, Moravec was not specifically interested in finding corners, just distinct regions in an image that could be used to register consecutive image frames. The Moravec operator is considered to be a corner detector because it defines interest points as points where there are large intensity variations in all directions. Moravec also defined the concept of "points of interest" in an image and concluded these interest points could be used to find matching regions in different images. Moravec in 1977 for his research involving the automatic navigation of a robot through a clustered environment.

One of the first operators for interest point detection was developed by Hans P. Corners, blobs, Harris corners, and differences of Gaussians of Harris corners are good features since they are repeatable and distinct. Robust correspondences are required in order to estimate the necessary transformation to align an image with the image it is being composited on. The set of images will have consistent exposure between frames to minimize the probability of seams occurring.įeature detection is necessary to automatically find correspondences between images. Additionally, the aspect ratio of a panorama image needs to be taken into account to create a visually pleasing composite.įor panoramic stitching, the ideal set of images will have a reasonable amount of overlap (at least 15–30%) to overcome lens distortion and have enough detectable features. In a non-ideal real-life case, the intensity varies across the whole scene, and so does the contrast and intensity across frames. Other major issues to deal with are the presence of parallax, lens distortion, scene motion, and exposure differences. Other reasons for seams could be the background changing between two images for the same continuous foreground. Since the illumination in two views cannot be guaranteed to be identical, stitching two images could create a visible seam. When multiple images exist in a panorama, techniques have been developed to compute a globally consistent set of alignments and to efficiently discover which images overlap one another.Ī final compositing surface onto which to warp or projectively transform and place all of the aligned images is needed, as are algorithms to seamlessly blend the overlapping images, even in the presence of parallax, lens distortion, scene motion, and exposure differences. Algorithms that combine direct pixel-to-pixel comparisons with gradient descent (and other optimization techniques) can be used to estimate these parameters.ĭistinctive features can be found in each image and then efficiently matched to rapidly establish correspondences between pairs of images. In order to estimate image alignment, algorithms are needed to determine the appropriate mathematical model relating pixel coordinates in one image to pixel coordinates in another.


VR PANORAMA STITCHER REGISTRATION
This sample image shows geometrical registration and stitching lines in panorama creation.
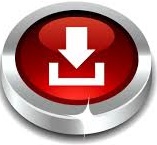